Joanna C. Chang
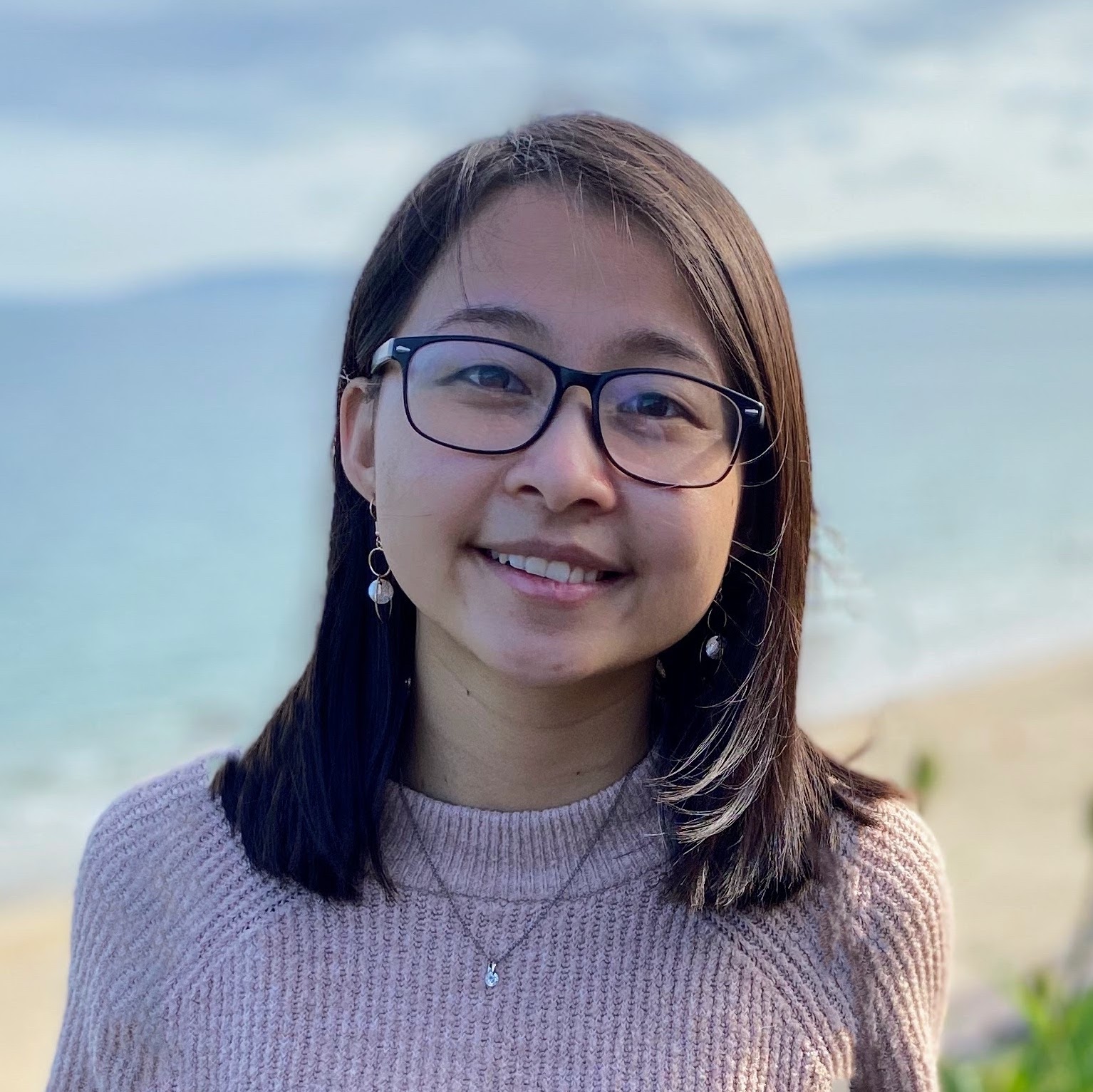
I’m a computational (and recently experimental) neuroscience PhD student at Imperial College London with Claudia Clopath and Juan Álvaro Gallego. My research focuses on modelling and analyzing neural population dynamics in the motor cortex to understand how we learn different motor skills.
Previously, I did my Master’s in Bioinformatics & Theoretical Systems Biology at Imperial and my undergraduate degree in Biology (with a Computer Science minor) at Pomona College in the US. Apart from research, I’m passionate about making science and technology more accessible to the general public.
projects
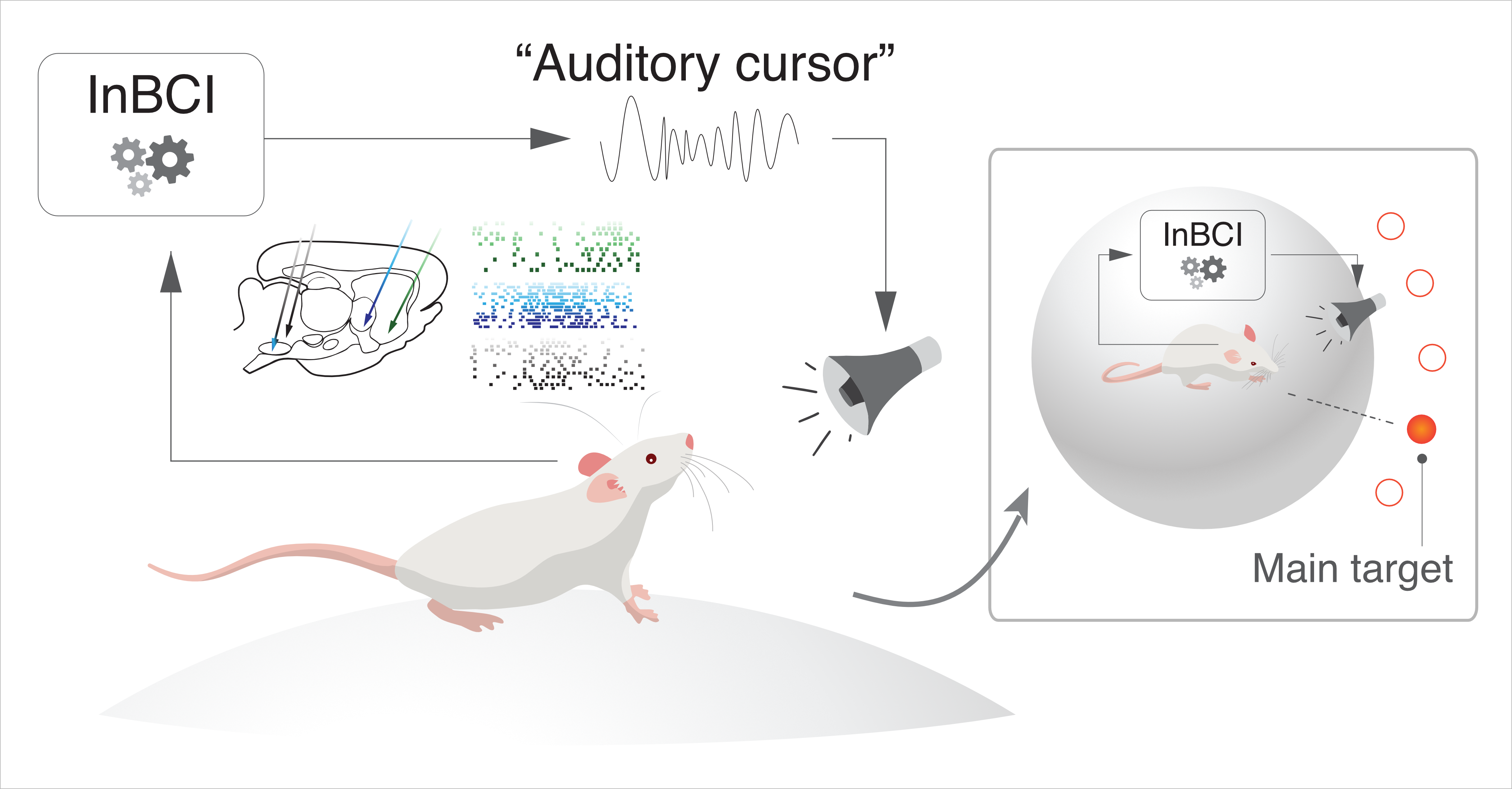
Subcortical BCI for motor control
Brain computer interfaces (BCIs) can help restore motor function by directly reading the user's intent from their brain. However, current BCIs lack the ability to provide stable control over skilled movements. To address this problem, we are building a BCI based on activity in subcortical nuclei of the basal ganglia, which are important for skilled and automatic movements. Currently, we are using neural population recordings from both cortex and basal ganglia to understand their contributions to motor control, along with behavioral recordings while mice perform both naturalistic and skilled behaviors.
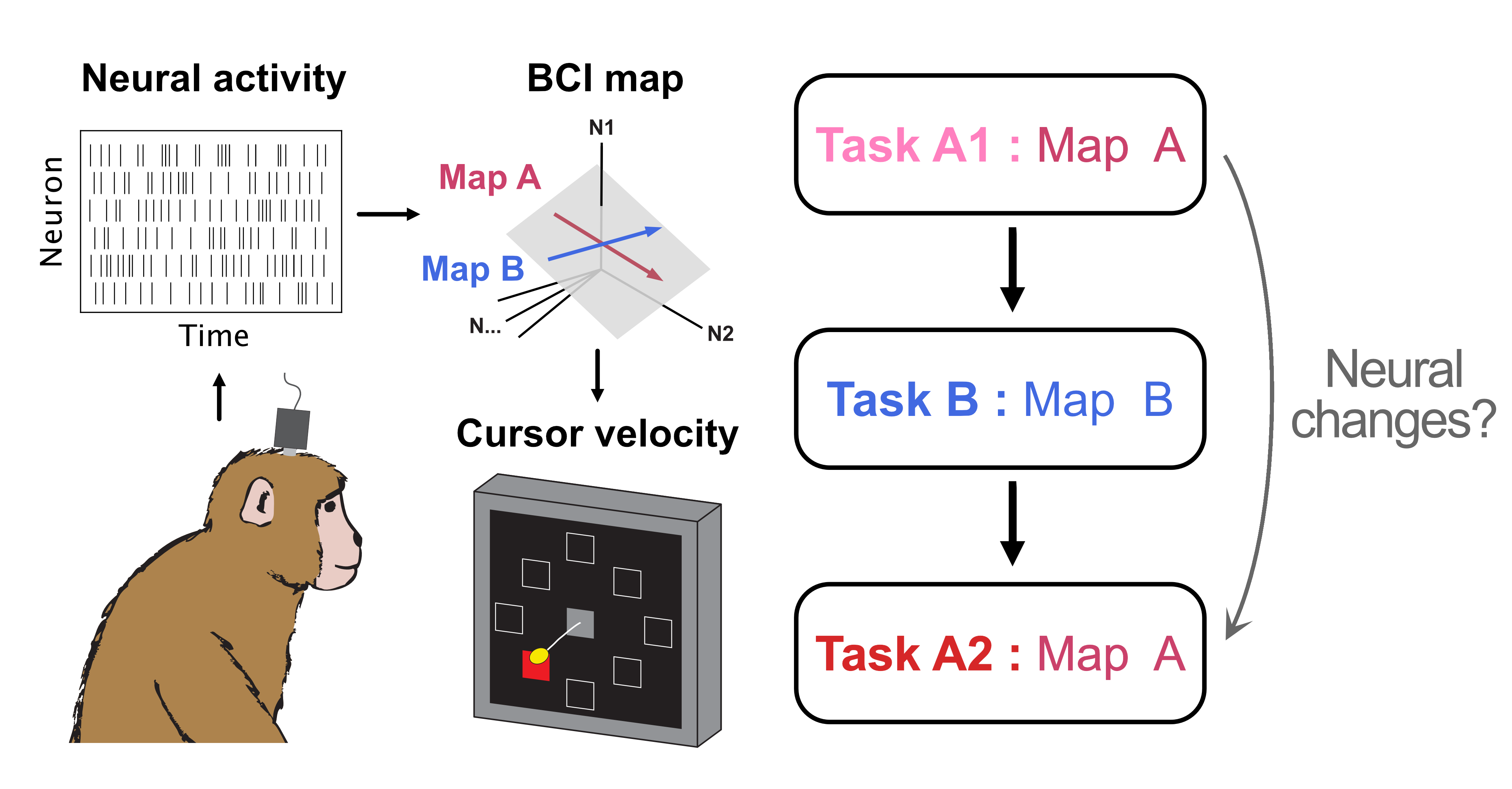
Memory traces arising from sequential learning
Animals can learn and perform many behaviors without interference. How do changes in neural activity during learning avoid interference with activity underlying similar behaviors? Recently, Losey et al (bioRxiv, 2022) examined neural activity of monkeys controlling a BCI, and saw that learning led to 'memory traces' of new tasks. To further understand these memory traces and their role in continual learning, we are modelling the same BCI setup using RNNs and examining how they arise under sequential learning.
Presented at: COSYNE '23, Bernstein '23
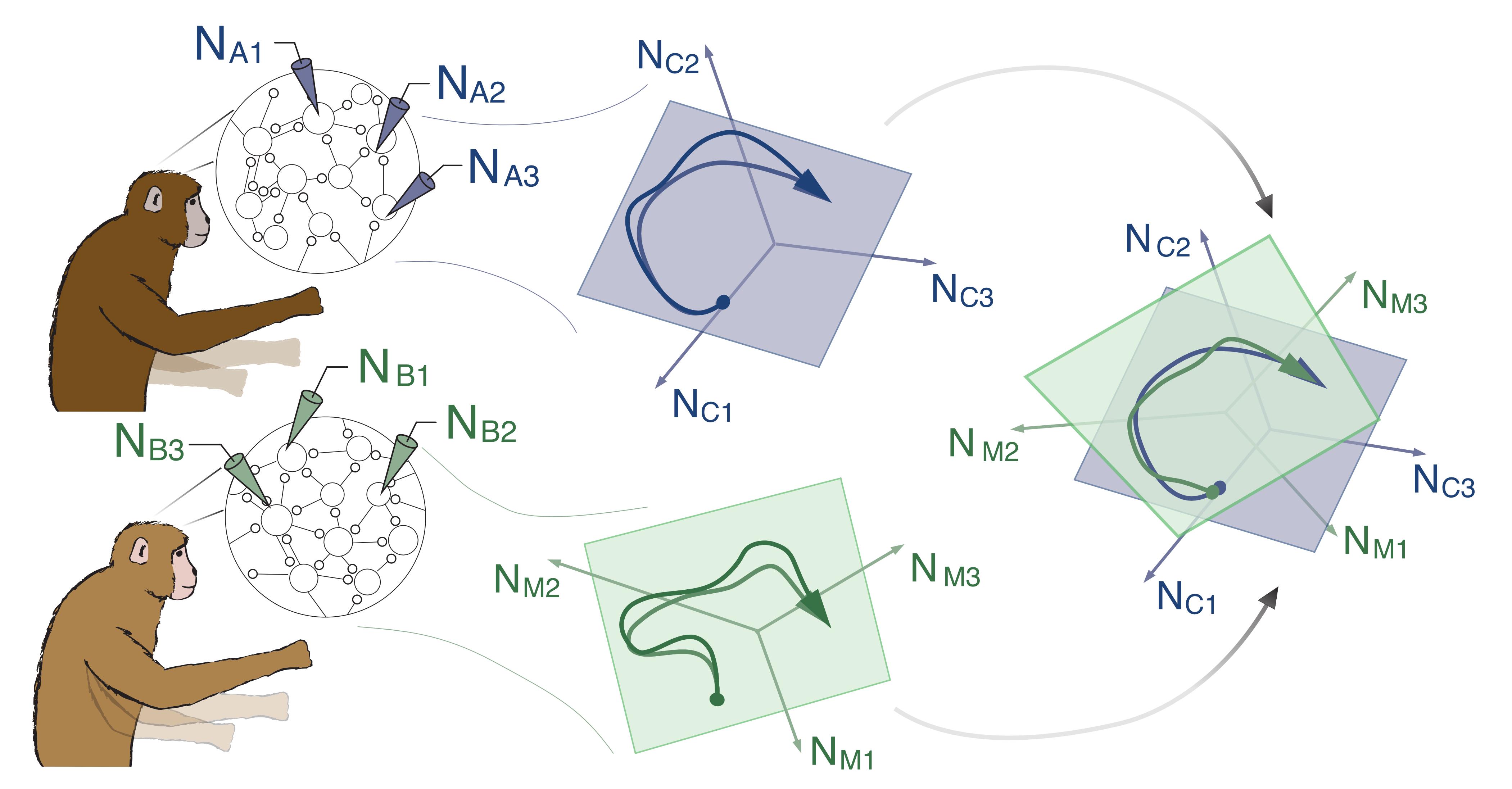
Aligned neural dynamics across animals
Different individuals of the same species perform similar adaptive behaviors. How does this happen when they have different brains with unique circuitries? Using data from three monkeys and four mice across three different behavioral tasks, we show that neural population latent dynamics are preserved across animals of the same species and may serve as the basis of species-specific behaviours.
Presented at: NCM '23, Mila
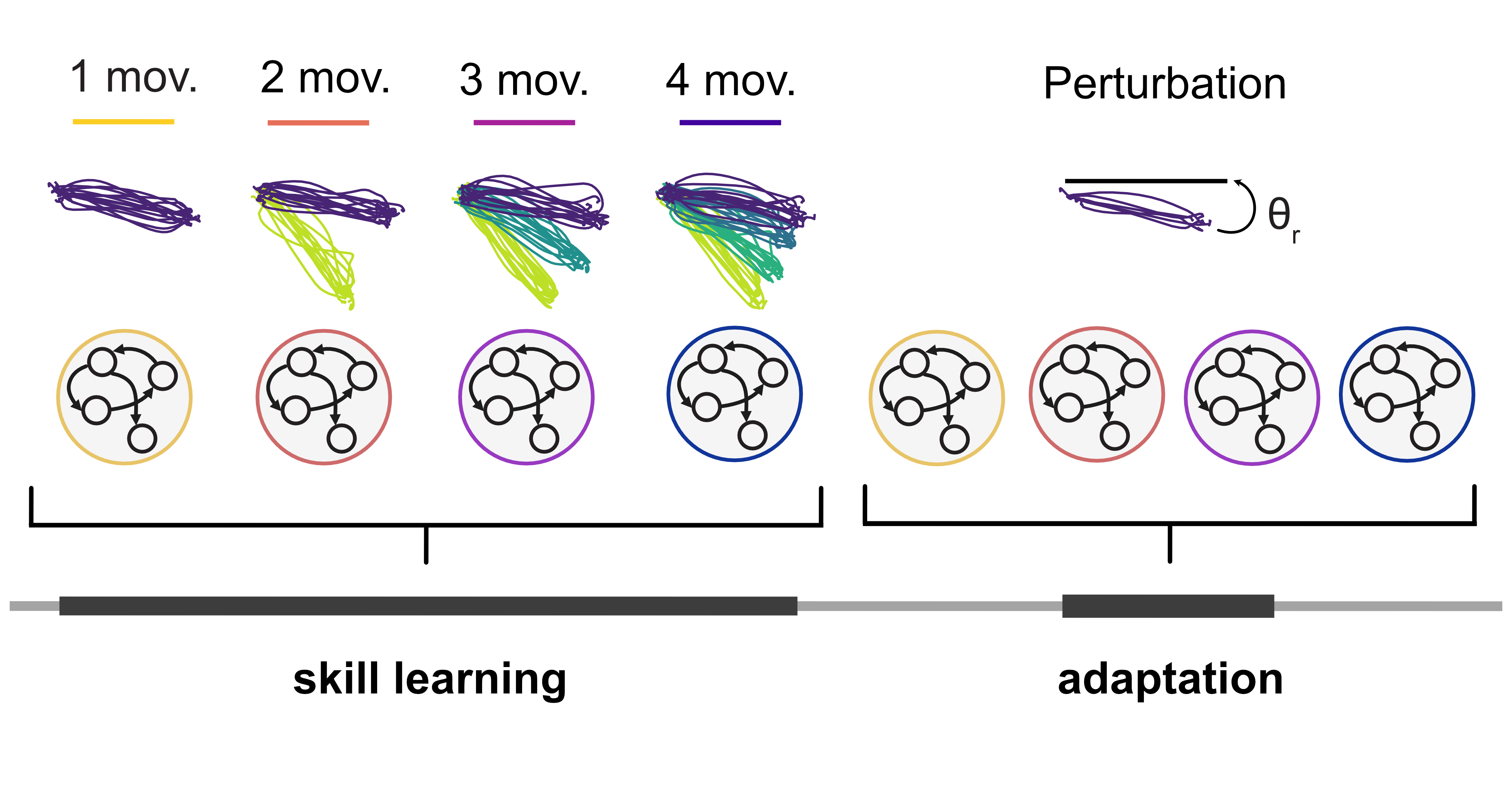
Neural space structure driving de novo learning and adaptation
We are constantly adapting learned behaviors to environmental changes, and what we know influences how easily we adapt—an experienced football player is better at maneuvering a muddy field than a novice player, for example. It is hard to study the neural basis for this phenomenon because it is impossible to track an animal’s lifelong learning experience, so we modeled it using RNNs instead. We found that different learning experiences led to qualitatively different neural representations, and the specific structure affected subsequent adaptation.
Presented at: COSYNE '22, NCM '22, COSYNE '23, Bernstein '23